Since the release of ChatGPT in November 2022, the GenAI
landscape has undergone rapid cycles of experimentation, improvement, and
adoption across a wide range of use cases. Applied to the software
engineering industry, GenAI assistants primarily help engineers write code
faster by providing autocomplete suggestions and generating code snippets
based on natural language descriptions. This approach is used for both
generating and testing code. While we recognise the tremendous potential of
using GenAI for forward engineering, we also acknowledge the significant
challenge of dealing with the complexities of legacy systems, in addition to
the fact that developers spend a lot more time reading code than writing it.
Through modernizing numerous legacy systems for our clients, we have found that an evolutionary approach makes
legacy displacement both safer and more effective at achieving its value goals. This method not only reduces the
risks of modernizing key business systems but also allows us to generate value early and incorporate frequent
feedback by gradually releasing new software throughout the process. Despite the positive results we have seen
from this approach over a “Big Bang” cutover, the cost/time/value equation for modernizing large systems is often
prohibitive. We believe GenAI can turn this situation around.
For our part, we have been experimenting over the last 18 months with
LLMs to tackle the challenges associated with the
modernization of legacy systems. During this time, we have developed three
generations of CodeConcise, an internal modernization
accelerator at Thoughtworks . The motivation for
building CodeConcise stemmed from our observation that the modernization
challenges faced by our clients are similar. Our goal is for this
accelerator to become our sensible default in
legacy modernization, enhancing our modernization value stream and enabling
us to realize the benefits for our clients more efficiently.
We intend to use this article to share our experience applying GenAI for Modernization. While much of the
content focuses on CodeConcise, this is simply because we have hands-on experience
with it. We do not suggest that CodeConcise or its approach is the only way to apply GenAI successfully for
modernization. As we continue to experiment with CodeConcise and other tools, we
will share our insights and learnings with the community.
GenAI era: A timeline of key events
One primary reason for the
current wave of hype and excitement around GenAI is the
versatility and high performance of general-purpose LLMs. Each new generation of these models has consistently
shown improvements in natural language comprehension, inference, and response
quality. We are seeing a number of organizations leveraging these powerful
models to meet their specific needs. Additionally, the introduction of
multimodal AIs, such as text-to-image generative models like DALL-E, along
with AI models capable of video and audio comprehension and generation,
has further expanded the applicability of GenAIs. Moreover, the
latest AI models can retrieve new information from real-time sources,
beyond what is included in their training datasets, further broadening
their scope and utility.
Since then, we have observed the emergence of new software products designed
with GenAI at their core. In other cases, existing products have become
GenAI-enabled by incorporating new features previously unavailable. These
products typically utilize general purpose LLMs, but these soon hit limitations when their use case goes beyond
prompting the LLM to generate responses purely based on the data it has been trained with (text-to-text
transformations). For instance, if your use case requires an LLM to understand and
access your organization’s data, the most economically viable solution often
involves implementing a Retrieval-Augmented Generation (RAG) approach.
Alternatively, or in combination with RAG, fine-tuning a general-purpose model might be appropriate,
especially if you need the model to handle complex rules in a specialized
domain, or if regulatory requirements necessitate precise control over the
model’s outputs.
The widespread emergence of GenAI-powered products can be partly
attributed to the availability of numerous tools and development
frameworks. These tools have democratized GenAI, providing abstractions
over the complexities of LLM-powered workflows and enabling teams to run
quick experiments in sandbox environments without requiring AI technical
expertise. However, caution must be exercised in these relatively early
days to not fall into traps of convenience with frameworks to which
Thoughtworks’ recent technology radar
attests.
Problems that make modernization expensive
When we began exploring the use of “GenAI for Modernization”, we
focused on problems that we knew we would face again and again – problems
we knew were the ones causing modernization to be time or cost
prohibitive.
- How can we understand the existing implementation details of a system?
- How can we understand its design?
- How can we gather knowledge about it without having a human expert available
to guide us? - Can we help with idiomatic translation of code at scale to our desired tech
stack? How? - How can we minimize risks from modernization by improving and adding
automated tests as a safety net? - Can we extract from the codebase the domains, subdomains, and
capabilities? - How can we provide better safety nets so that differences in behavior
between old systems and new systems are clear and intentional? How do we enable
cut-overs to be as headache free as possible?
Not all of these questions may be relevant in every modernization
effort. We have deliberately channeled our problems from the most
challenging modernization scenarios: Mainframes. These are some of the
most significant legacy systems we encounter, both in terms of size and
complexity. If we can solve these questions in this scenario, then there
will certainly be fruit born for other technology stacks.
The Architecture of CodeConcise
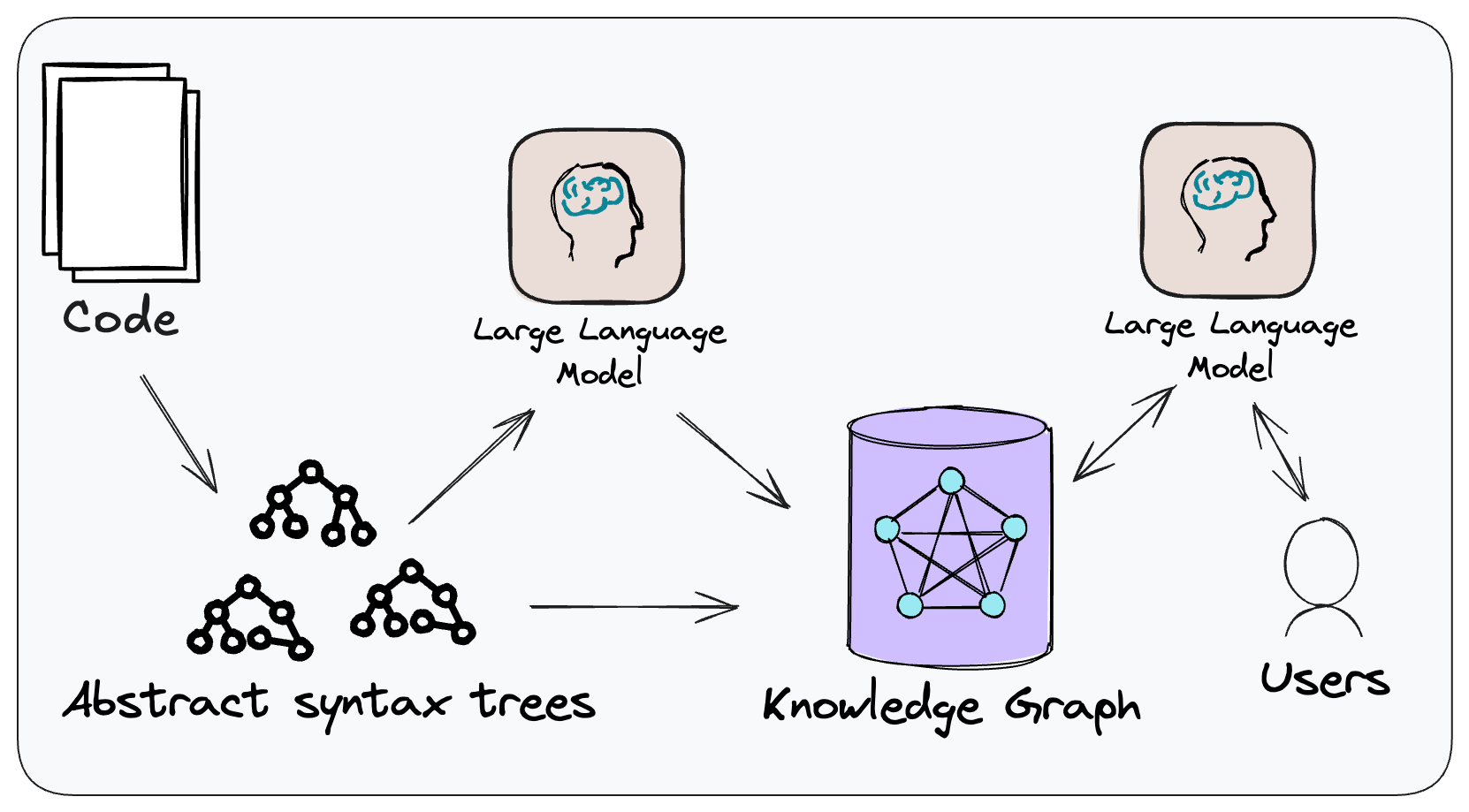
Figure 1: The conceptual approach of CodeConcise.
CodeConcise is inspired by the Code-as-data
concept, where code is
treated and analyzed in ways traditionally reserved for data. This means
we are not treating code just as text, but through employing language
specific parsers, we can extract its intrinsic structure, and map the
relationships between entities in the code. This is done by parsing the
code into a forest of Abstract Syntax Trees (ASTs), which are then
stored in a graph database.

Figure 2: An ingestion pipeline in CodeConcise.
Edges between nodes are then established, for example an edge might be saying
“the code in this node transfers control to the code in that node”. This process
does not only allow us to understand how one file in the codebase might relate
to another, but we also extract at a much granular level, for example, which
conditional branch of the code in one file transfers control to code in the
other file. The ability to traverse the codebase at such a level of granularity
is particularly important as it reduces noise (i.e. unnecessary code) from the
context provided to LLMs, especially relevant for files that do not contain
highly cohesive code. Primarily, there are two benefits we observe from this
noise reduction. First, the LLM is more likely to stay focussed on the prompt.
Second, we use the limited space in the context window in an efficient way so we
can fit more information into one single prompt. Effectively, this allows the
LLM to analyze code in a way that is not limited by how the code is organized in
the first place by developers. We refer to this deterministic process as the ingestion pipeline.
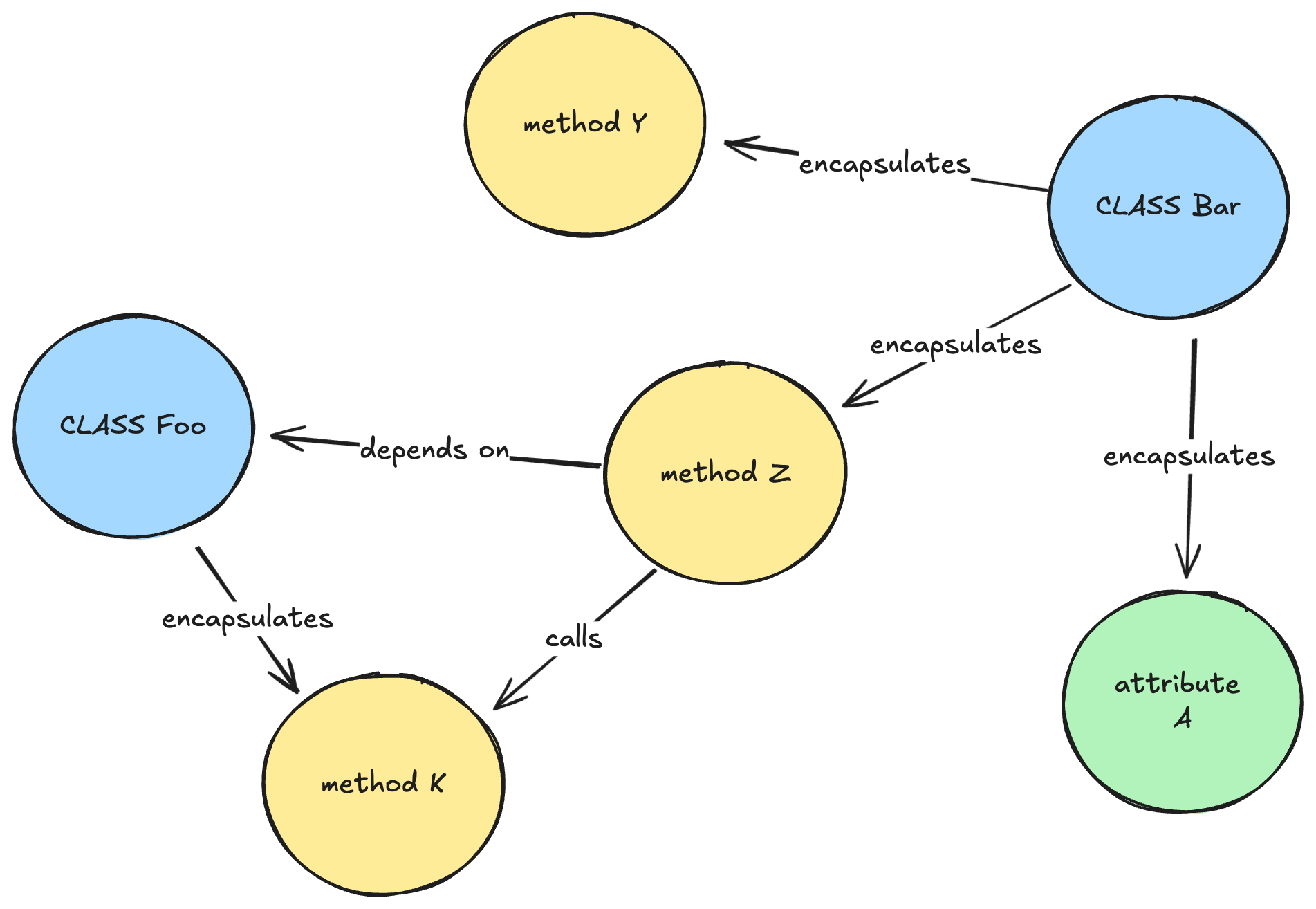
Figure 3: A simplified representation of how a knowledge graph might look like for a Java codebase.
Subsequently, a comprehension pipeline traverses the graph using multiple
algorithms, such as Depth-first Search with
backtracking in post-order
traversal, to enrich the graph with LLM-generated explanations at various depths
(e.g. methods, classes, packages). While some approaches at this stage are
common across legacy tech stacks, we have also engineered prompts in our
comprehension pipeline tailored to specific languages or frameworks. As we began
using CodeConcise with real, production client code, we recognised the need to
keep the comprehension pipeline extensible. This ensures we can extract the
knowledge most valuable to our users, considering their specific domain context.
For example, at one client, we discovered that a query to a specific database
table implemented in code would be better understood by Business Analysts if
described using our client’s business terminology. This is particularly relevant
when there is not a Ubiquitous
Language shared between
technical and business teams. While the (enriched) knowledge graph is the main
product of the comprehension pipeline, it is not the only valuable one. Some
enrichments produced during the pipeline, such as automatically generated
documentation about the system, are valuable on their own. When provided
directly to users, these enrichments can complement or fill gaps in existing
systems documentation, if one exists.
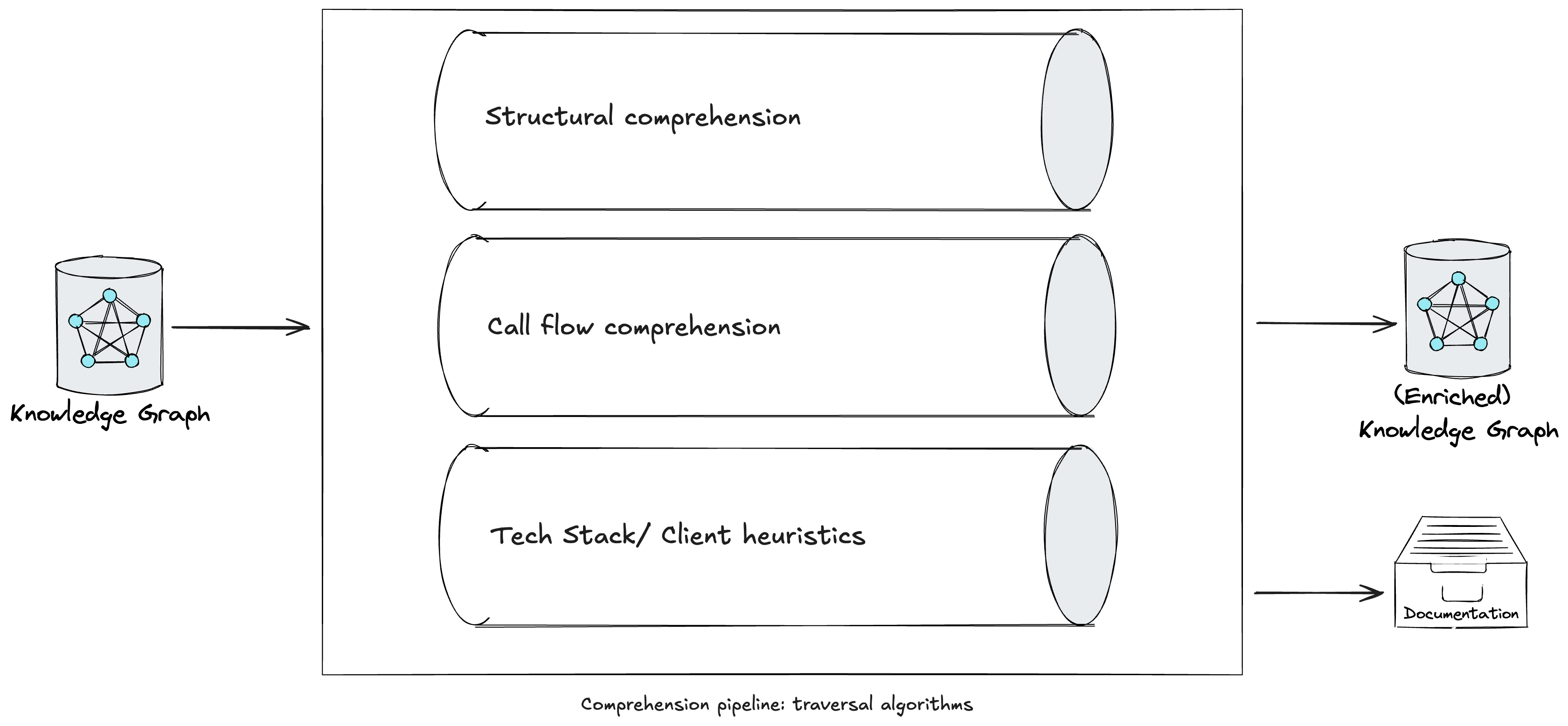
Figure 4: A comprehension pipeline in CodeConcise.
Neo4j, our graph database of choice, holds the (enriched) Knowledge Graph.
This DBMS features vector search capabilities, enabling us to integrate the
Knowledge Graph into the frontend application implementing RAG. This approach
provides the LLM with a much richer context by leveraging the graph’s structure,
allowing it to traverse neighboring nodes and access LLM-generated explanations
at various levels of abstraction. In other words, the retrieval component of RAG
pulls nodes relevant to the user’s prompt, while the LLM further traverses the
graph to gather more information from their neighboring nodes. For instance,
when looking for information relevant to a query about “how does authorization
work when viewing card details?” the index may only provide back results that
explicitly deal with validating user roles, and the direct code that does so.
However, with both behavioral and structural edges in the graph, we can also
include relevant information in called methods, the surrounding package of code,
and in the data structures that have been passed into the code when providing
context to the LLM, thus provoking a better answer. The following is an example
of an enriched knowledge graph for AWS Card
Demo,
where blue and green nodes are the outputs of the enrichments executed in the
comprehension pipeline.
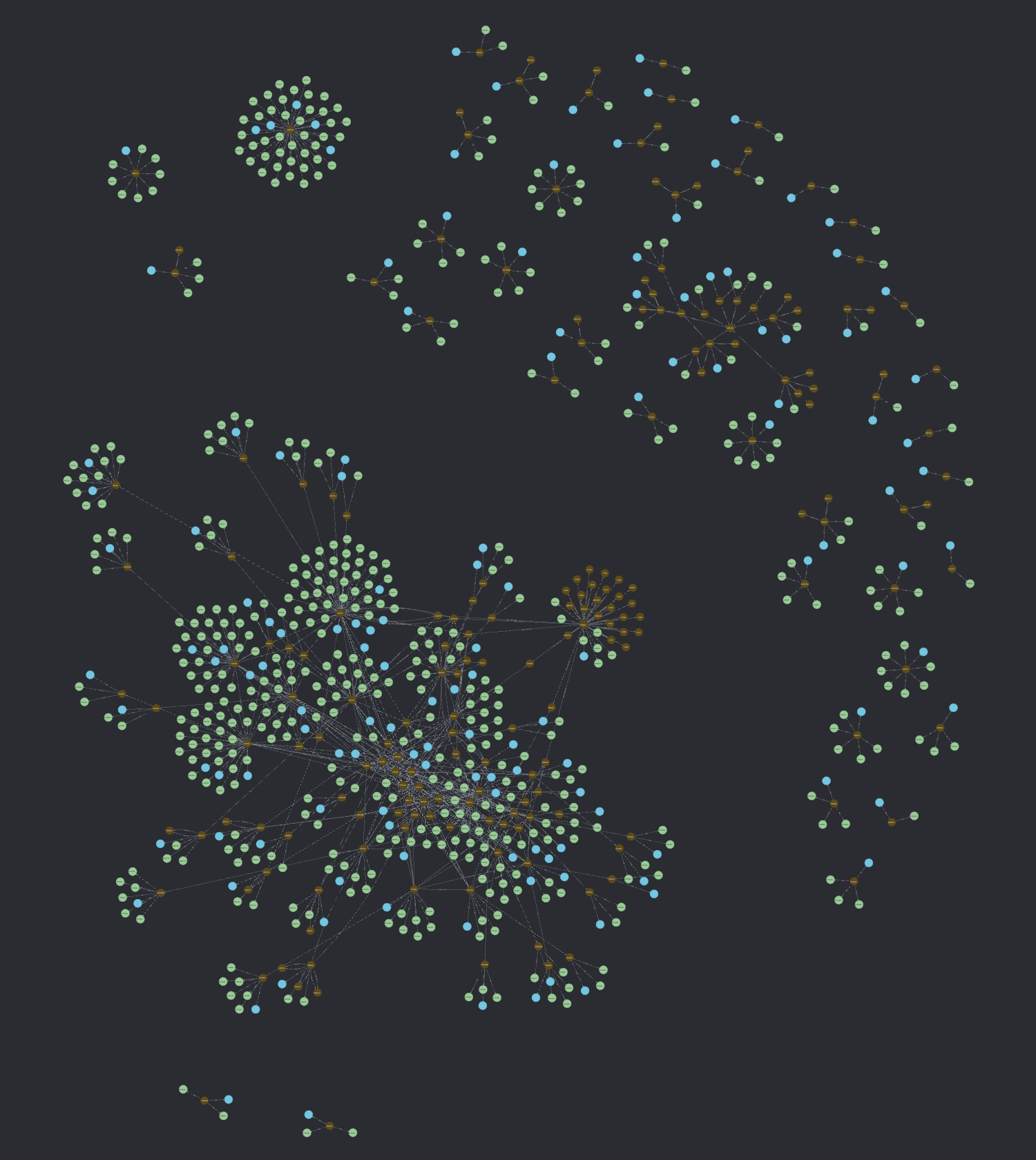
Figure 5: An (enriched) knowledge graph for AWS Card Demo.
The relevance of the context provided by further traversing the graph
ultimately depends on the criteria used to construct and enrich the graph in the
first place. There is no one-size-fits-all solution for this; it will depend on
the specific context, the insights one aims to extract from their code, and,
ultimately, on the principles and approaches that the development teams followed
when constructing the solution’s codebase. For instance, heavy use of
inheritance structures might require more emphasis on INHERITS_FROM
edges vs
COMPOSED_OF
edges in a codebase that favors composition.
For further details on the CodeConcise solution model, and insights into the
progressive learning we had through the 3 iterations of the accelerator, we
will soon be publishing another article: Code comprehension experiments with
LLMs.
In the subsequent sections, we delve deeper into specific modernization
challenges that, if solved using GenAI, could significantly impact the cost,
value, and time for modernization – factors that often discourage us from making
the decision to modernize now. In some cases, we have begun exploring internally
how GenAI might address challenges we have not yet had the opportunity to
experiment with alongside our clients. Where this is the case, our writing is
more speculative, and we have highlighted these instances accordingly.